- The Vision, Debugged;
- Posts
- Kuwain 1.5B: Teaching an English AI to Speak Arabic Without Starting From Scratch
Kuwain 1.5B: Teaching an English AI to Speak Arabic Without Starting From Scratch
PLUS: We tested AI's location detection skillsāthe results are shocking š²

Howdy Vision Debuggers!šµļø
This week, Spark and Trouble are tuning their radars to pick up whispers from new corners of the AI world, and trust us, the signal is strong and full of surprises. š”āØ
Hereās a sneak peek into todayās edition š
Arabic-English AI breakthrough slashes training costs by 70%
What if Einstein and Sun Tzu joined your brainstorm? Try todayās Fresh Prompt
5 powerful AI tools that will blow your mind
AI Can Guess Your Photo's Location (Even Without Landmarks!) - see how!
Time to jump in!š
PS: Got thoughts on our content? Share 'em through a quick survey at the end of every edition It helps us see how our product labs, insights & resources are landing, so we can make them even better.

Hot off the Wires š„
We're eavesdropping on the smartest minds in research. 𤫠Don't miss out on what they're cooking up! In this section, we dissect some of the juiciest tech research that holds the key to what's next in tech.ā”
Remember learning a second language in school? How you'd struggle with new vocabulary, grammar rules, and pronunciations while trying not to forget your native language? For most of us, becoming truly bilingual required years of practice and immersion.
Well, AI researchers faced a similar challenge - how do you teach an English-speaking AI model to understand and generate Arabic without making it "forget" its English abilities or breaking the bank on training costs?
The answer comes in the form of "Kuwain 1.5B," an innovative approach that's changing how we make language models multilingual.
Kuwain is a compact 1.5 billion-parameter bilingual Arabic-English model that achieves impressive performance in both languages - but what makes it truly groundbreaking is how it was created: through a technique called "language injection" that's both cost-effective and surprisingly efficient.
So, whatās new?
Most large language models (LLMs) today suffer from an English-centric bias. Models like GPT-4, Claude, and even smaller open-source alternatives are primarily trained on English data, making them less effective for languages with distinct scripts and rich morphological structures like Arabic. This creates a significant gap in AI capabilities for billions of non-English speakers worldwide.
Traditional approaches to solving this problem are prohibitively expensive. Building a fully bilingual model from scratch requires massive amounts of data and computing power.
Even fine-tuning existing models on new languages can increase costs by up to 68% due to inefficient tokenization and the need to retrain all parameters.
Kuwain takes a radically different approach. Rather than retraining an entire model, the researchers "injected" Arabic capabilities into TinyLlama (a 1.1B parameter English model) by:
Freezing the original model parameters
Strategically adding just 8 new transformer layers
Expanding the tokenizer's vocabulary to include 26,000 Arabic tokens
The result? A model that achieves an impressive 8% uplift on Arabic benchmarks while maintaining (and slightly improving) its English performance - all at 70% lower training cost compared to traditional methods.
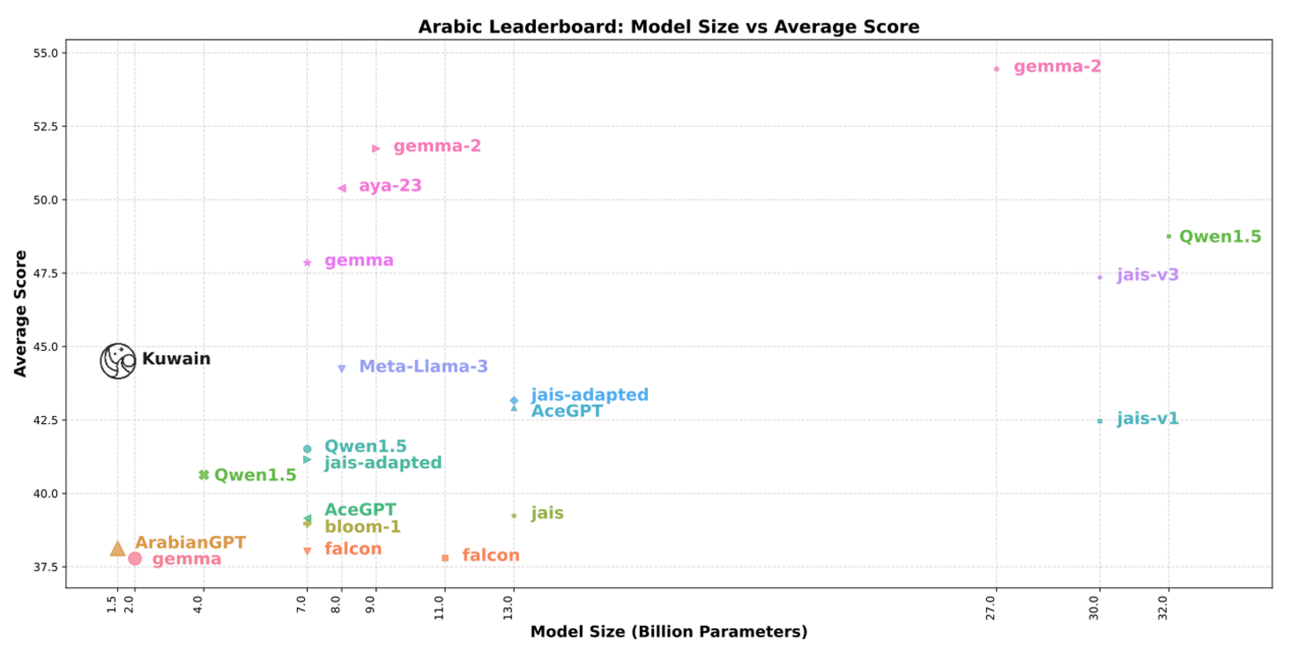
Model performance vs size visualization on the Arabic NLP benchmark (source: Kuwain paper)
Forging the fundamentals
Letās refresh some key concepts before diving into the detailsā¦
Transformer Model: A type of neural network architecture that revolutionized AI by using "attention mechanisms" to process text all at once instead of sequentially. Think of it like a reader who can see and connect relationships between all words in a sentence simultaneously rather than reading word by word. Most modern language models (like GPT and BERT) are built on this architecture.
Tokenizer: The tool that breaks text into smaller pieces (tokens) that a language model can understand. Imagine a translator who first splits foreign sentences into familiar chunks before translating. Tokenizers might break words into whole words, parts of words, or even individual characters, depending on the language and specific AI system.
Catastrophic Forgetting: When an AI model loses previously learned knowledge while learning new information. It's like a student who completely forgets algebra while learning calculus. This is particularly problematic when adapting models from one language to another, as the model might lose its skills in the original language.
Expansion Ratio: A measure of how efficiently a tokenizer breaks down text, calculated as the ratio of tokenized length to original word count. A lower ratio is generally better. Think of it as a compression rate - if English text uses 100 tokens for 50 words (ratio 2.0) while Arabic uses 150 tokens for 50 words (ratio 3.0), the tokenization is less efficient for Arabic.
Under the hoodā¦
The magic of Kuwain's approach lies in three key innovations:
1. Selective Layer Extension
Instead of adding new layers all at once, the researchers carefully distributed 8 new "identity blocks" at non-uniform intervals throughout the model. These blocks are initialized to have no effect at first (acting as an identity function), allowing them to gradually learn Arabic-specific patterns without disrupting the existing English knowledge.

Extension of model layers, where newly added layers were trainable while the original layers remained frozen (source: Kuwain paper)
of model layers, where newly added layers were trainable while the original layers remained frozen.
Think of it like inserting new specialized teachers into specific points of a student's educational journey, rather than completely replacing the entire faculty. Each new teacher builds on what previous teachers taught while adding new language skills.
Some key findings that the researchers had during experimentation include:
⤠Avoid stacking new layers consecutivelyāleads to instability
⤠Keep the final encoder layer trainableāfreezing it causes volatility
⤠Best configuration: 8 layers distributed non-uniformly yields stable training and top performance
2. Targeted Vocabulary Expansion
Languages use different character sets and word formations. The researchers trained a custom Arabic-aware tokenizer that generated 26,000 new tokens specifically for Arabic, then merged these with TinyLlama's original 28,000 tokens (making it a total of 54,000 tokens).

Vocabulary expansion by adding new Arabic tokens to the tokenizer (source: Kuwain paper)
This balanced approach achieves what they call an "expansion ratio" of 2.30 - essentially a measure of tokenization efficiency (lower expansion ratio = better tokenization efficiency).
For comparison, previous Arabic models like AraBERT had less efficient ratios of 2.51 despite using twice as many Arabic tokens (~54,000).
3. Data Anchoring
Perhaps most surprisingly, the researchers found that mixing just 20% of the original English data with the new Arabic training data was sufficient to maintain English performance. This "anchoring" technique drastically cuts data needs compared to prior work that required 50% or more of the original language data.
The training process itself was remarkably efficient, using 8 A100 GPUs for just 3 epochs with an effective batch size of 1 million tokens. By freezing the base layers and only training the new injected layers, Kuwain avoids the dreaded "catastrophic forgetting" problem that plagues many language adaptation approaches.
Results speak louder than wordsā¦
To see how well Kuwain works, researchers tested it against established benchmarks for both Arabic and English. The results were impressive:
Arabic Performance: Kuwain's average score jumped from 36.95 to 44.49 - an approximately 8% absolute gain over the base TinyLlama model on key tasks like BoolQ, ARC, and HellaSwag. This puts it on par with much larger models (7B to 70B parameters) on the Open Arabic LLM Leaderboard.
English Performance: Rather than degrading English capabilities (as often happens with continual pre-training approaches), Kuwain maintained or slightly improved its English performance, with average scores increasing from 52.99 to 53.28.
For comparison, a "naive" approach that simply expanded the vocabulary and continued pre-training without layer extension showed similar Arabic gains but suffered severe English degradation (average English scores dropped from 52.99 to 46.85).
The researchers even created a fine-tuned version called "Lahajawi" that excels in Arabic cross-dialect translation, demonstrating that Kuwain serves as an excellent foundation for downstream Arabic NLP tasks.
Why does this matter?
Kuwain's language injection approach isn't just an academic curiosity - it has profound implications for making AI more accessible and inclusive:
Democratizing Multilingual AI: By reducing training costs by 70%, this approach brings multilingual AI capabilities within reach of smaller labs and organizations with limited GPU budgets. No longer is multilingual AI the exclusive domain of tech giants with massive computing resources.
Preserving While Expanding: The technique demonstrates that models can learn new languages without sacrificing their original capabilities - a challenging balance that previous approaches struggled to achieve.
Scalable Localization: While this research focused on Arabic, the same pipeline could be applied to inject capabilities for other underrepresented languages with distinct scripts and morphological structures, from Thai to Amharic to Telugu.
Practical Applications: From customer service chatbots that can seamlessly switch between English and Arabic to educational tools that help with language learning, Kuwain's approach enables a new generation of truly bilingual AI assistants.
In a world where over 420 million people speak Arabic as their first language, making AI systems accessible in this language isn't just good business. It's a matter of digital equality. Kuwain represents a significant step toward AI systems that can serve diverse linguistic communities without requiring prohibitive investments.
What's your take? Do you think this selective language injection approach could help bridge the language gap in AI for other underrepresented languages?
Share your thoughts with Spark & Trouble.
Wish to explore Kuwain further?
⤠Check out the research paper
⤠Play around with the GitHub repo

10x Your Workflow with AI š
Work smarter, not harder! In this section, youāll find prompt templates š & bleeding-edge AI tools āļø to free up your time.
Fresh Prompt Alert!šØ
Ever wish you could time-travel and grab coffee with history's greatest thinkers? This week's prompt brings Einstein, Sun Tzu, and other brilliant minds straight to your brainstorming sessions!
Whether you're stuck on a product roadmap or an ethical dilemma, these virtual mentors offer wildly different perspectives to unlock fresh insights. It's like having the ultimate advisory board in your pocket, minus the time machine and awkward small talk.
Give it a try and watch your challenges transform into opportunities! š
Act as a panel of historyās greatest minds, including Leonardo da Vinci, Marie Curie, Socrates, Einstein, Sun Tzu, and Maya Angelou.
Iāll share a modern-day idea, challenge, or decision. For each thinker, give unique feedback based on their worldview and expertise.
For example, Einstein might focus on systems and imagination, while Sun Tzu would assess the strategic angle.
Let them offer contrasting opinions if it deepens the insight. Then, summarize the most valuable takeaways and end with one thought-provoking question I should reflect on.
Hereās my challenge: [Insert your idea, goal, or dilemma]
Discover new capabilities in tools you already love ā with our Fresh Prompt Alerts.
5 AI Tools You JUST Can't Miss š¤©
š§ ScopyMe: Craft business strategies in Minutes
š» Tempo Labs: Build React apps 10X faster with AI
š¤ GenFuse AI: Automate any work with AI agents (no technical skills required)
š„ MenuExplainer: Snap a photo of any menu, any language & get the breakdown of each dish with images
š¼ļø Graficto: Create powerful smart infographics and visuals without any design skills
Want more? Check out our AI Tools Showcase for 200+ handpicked AI toolsā¦

Spark 'n' Trouble Shenanigans š
Ever wondered if an AI could guess where you snapped a photo, even without any famous landmarks?
Well, Spark & Trouble went down that rabbit hole this week... and letās just say, itās both wildly cool and mildly dystopian.
Armed with OpenAIās new o3 and o4-mini models, we fed them some sneaky photos ā and boom ā they Sherlock-ed their way through random hills, blurry license plates, and cafĆ© patios to almost pinpoint the locations.
(Yes, Trouble even tested it with a nostalgic throwback from his IIT Bombay days... and the model nearly nailed it! Check out the conversation here)

The original photo

Response from o4-mini - right on target!
It's equally fascinating and slightly terrifying how these models connect visual dots that most humans would miss.
Want to test this digital detective yourself? Try feeding your vacation photos to o3, o4-mini, or Claude and see if they can nail your whereabouts, then share your experiences with us!

Well, thatās a wrap! Until then, | ![]() |

Reply