- The Vision, Debugged;
- Posts
- Worried About Fake Audio Scams? This AI Research Can Help
Worried About Fake Audio Scams? This AI Research Can Help
PLUS: Strategic Moves Behind the Nvidia's Meteoric Success

Howdy fellas!
Brace yourselves for another insight-packed edition of āThe Vision, Debuggedā, where Spark & Trouble are about to take you on a wild ride through the latest AI research that's turning the world on its head.
But that's not all ā they've got the tools and prompts to help you harness this power and soar to new heights.
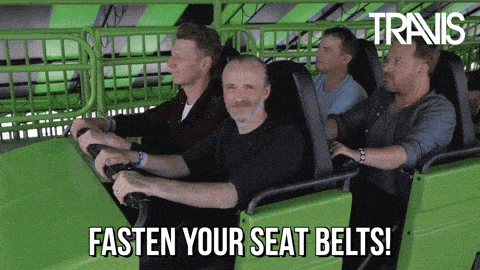
Gif by travisband on Giphy
Hereās a sneak peek into todayās edition š
Learn about the latest AI research that detects audio deep fakes with ferocious accuracy
3 AI tools for peak productivity, that you JUST cannot miss!
5 strategy lessons to learn from Nvidia's meteoric rise in this AI gold rush
Time to jump in!š
PS: Got thoughts on our content? Share 'em through a quick survey at the end of every edition It helps us see how our product labs, insights & resources are landing, so we can make them even better.

Hot off the Wires š„
We're eavesdropping on the smartest minds in research. 𤫠Don't miss out on what they're cooking up! In this section, we dissect some of the juiciest tech research that holds the key to what's next in tech.ā”
Imagine getting a frantic call from "Mom" begging for money because she's been arrested. But is it really Mom?

AI-based audio fraud and scams are on the rise (source: veille-cyber.com)
If you've been following the news lately, you've probably heard about the alarming rise of AI-based audio fraud and scams, particularly in India and other countries. Scammers are now using advanced audio deepfake technology to generate convincingly realistic synthetic voices of loved ones, tricking victims into paying ransoms under the pretence of a kidnapping or legal trouble. Scary stuff!
This underscores the urgent need for robust audio deepfake detection capabilities. Enter the brilliant minds at Ping An Technology (Shenzhen) Co. Ltd., who have developed a groundbreaking approach called Retrieval-Augmented Audio Deepfake Detection to combat this rapidly evolving threat.
So, whatās new?
Deepfake technology is like a crafty criminal ā it's constantly evolving. The bad news? Traditional deepfake detection methods are struggling to keep up. Existing frameworks often rely on a single detection model, and much like a lone security guard against a team of thieves, they can be overwhelmed.
To overcome this issue, researchers took inspiration from an interesting source: RAG (Retrieval Augmented Generation)! Since RAG has shown promising results in several retrieval-based question-answering systems using LLMs and is popularly deployed across several products, these folks thought, "Why not adapt this for audio deepfake detection?".
And so, their quest for Retrieval Augmented Detection (RAD) began!

E2E audio deep fake detection framework, inspired by RAG (source: RAD paper)
Under the hoodā¦
Now, before we dive into the nitty-gritty of this ray of hope, letās dive into some key concepts:
RAG (Retrieval Augmented Generation): A technique that combines a pre-trained large language model (LLM) with an information retrieval (IR) system over a vast knowledge database. Given a query, the IR system is used to retrieve most similar document snippets to the query & using these, the LLM constructs its final answer. Not only does this boost performance, but the retrieved evidence also justifies the model's decisions. Think of it as a detective who not only has good intuition but can also consult a vast database of past cases.
MFA (Multi-Fusion Attention): Technically, this method analyzes different features of multiple inputs simultaneously, then combines the results for a more comprehensive understanding. This is different from standard āattentionā, which focuses on a single input and highlights important parts within it. Imagine you're reading a document with images and charts. Regular attention in AI is like focusing on one sentence at a time. MFA is like reading the text, looking at the images, and checking the charts all at once, understanding how they all connect to give you the full picture.
EER (Equal Error Rate): For our techie friends, it's the point where a model's false positive and false negative rates intersect. For the rest of us, think of it as the sweet spot balancing incorrect predictions. Lower EER values indicating better performance.
Why bring up EER? Well, this innovation achieves an impressive 0.40% EER on a challenging audio deepfake detection benchmark! But we're getting ahead of ourselvesā¦
At the heart of this breakthrough lies a powerful trio: an Audio Feature Extractor, a Knowledge Retrieval Database, and a Detection Model. Letās take a look at how these components function together:

Detailed view of the RAD pipeline (source: RAD paper)
Audio Feature Extractor:
This part of the system first analyses the audio sample using Microsoftās state-of-the-art multilingual speech encoder model called WavLM. It captures both high-level and low-level acoustic details from the input audio, converting them into vector representations.
Note: Since WavLM was originally trained only on real audio (not fake/generated samples), so this feature extractor was finetuned by connecting it to a model for classifying a dataset of audio samples into real or fake.
For inference, this attachment is removed & the fineuned WavLM model is used as is.
Knowledge Retrieval Database:
This is where the inspiration from RAG comes in. In RAG applications, documents are segmented into snippets, which are converted into vector embedding & stored in a database for retrieval. In this case, real audio samples are segmented & processed by the feature extractor into long latent embeddings. These long feature representations are:
Temporally averaged into dense āembeddingsā (to be used for lookup)
Subjected to temporal speedup to compress them into āshort featuresā (to be used for detection)
The embeddings are mapped to corresponding short features.
WavLM feature extractor has multiple layers, so these embeddings extracted from each layer from WavLM are stored in a separate vector DB, for efficient retrieval (think of this as a sharded database).
During inference, the sample undergoes the same transformation into an embedding. A similarity search is performed across the databases to retrieve the top 10 most comparable embeddings of real audio, and their corresponding short features are returned to help in the detection process.
Detection Model:
The sample to be detected is compared against the retrieved similar real samples using a Multi-Fusion Attention (MFA) classifier. Internally, it uses something called the āattentive statistic poolingā mechanism to form intermediate representations for the sample to be detected & retrieved samples. It combines and compares information across different layers and timesteps to make the final classification. By paying close attention to the most important details, this MFA classifier can determine with impressive accuracy whether the audio is real or a deepfake.

Architecture of detection model using MFA (source: RAD paper)
Why does all this matter?
This new detection method has been tested with competitive, real-world datasets and achieved an impressive EER of less than 0.4% on some datasets!
To ensure broad applicability, the model was trained on ASVspoof datasets containing samples which include various spoofing algorithms (both seen and unseen during training). The researchers' aim? A future-proof model that generalizes to unseen synthesis techniques.
The results demonstrate the model's effectiveness and superiority in detecting both known and unknown audio deepfake generation techniques.
This cutting-edge research has immense potential:
Enhanced Security: Protects against fraud and misinformation by reliably detecting audio deepfakes.
Trust in Communication: Ensures the authenticity of digital audio, fostering trust.
Media Integrity: Maintains the credibility of media by identifying manipulated content.
Legal Evidence: Assists in verifying audio evidence in legal proceedings.
Spark and Trouble are thrilled by this groundbreaking work! We can't wait to see how it evolves and is applied in real products to help create a safer, more trustworthy digital world.
In fact, Truecaller recently announced that it can now detect if the caller is using AI to prevent AI call scams. Chances are, it might be using similar tech under the hood š§
Stay tuned for more hot-off-the-wires innovations!
Key Takeaways for Researchers & Data Scientists
(Screenshot this!)
Challenge Single-Model Detection: Consider limitations of such models in complex settings and explore ensemble methods for better results.
Creativity Sparks Innovation: Borrowing inspiration from existing research in not-so-related domains and augment it for your use cases could work wonders if you are able to draw reasonable parallels between them, demonstrating your creative thinking in data science.
Focus on Adaptability: Train models on diverse datasets with techniques that can make them robust enough to deal with previously unseen data.

10x Your Workflow with AI š
Work smarter, not harder! In this section, youāll find prompt templates š & bleeding-edge AI tools āļø to free up your time.
Fresh Prompt Alert!šØ
Chasing your dream career can be exhilarating yet daunting. Many stumble along the path, unsure of the pitfalls and detours. But fret not!
This prompt by Spark & Trouble will guide you through the common mistakes, providing a comprehensive roadmap to your desired profession, complete with time estimates and top-notch learning resources.
Let's craft your career journey together!
Could you outline the common pitfalls individuals encounter while pursuing their dream job of [Insert desired career here]?
Please provide a comprehensive, step-by-step guide to help circumvent these mistakes, complete with a detailed career roadmap that includes approximate durations for each stage.
Additionally, could you recommend the most effective learning resources available for this career path?
3 AI Tools You JUST Can't Miss š¤©

Spark 'n' Trouble Shenanigans š

When everyone digs for gold, sell shovels (source: Reddit)
By now, most of you are familiar with this meme doing the rounds on the internet ā āWhen everyone digs for gold, sell shovels.ā
That's basically generative AI right now. NVIDIA's stacking gold by supplying the tools, while the big guys like Microsoft, Google, and Meta are fighting over who gets to dig the most!
Well, here are some tips to really learn from Nvidia as it consolidates its place in todayās AI ecosystem:
Embrace Emerging Technologies with Confidence
Build a Strong Product Portfolio
Forge Strategic Partnerships
Navigate Technological Challenges with Agility
Stay Ahead of the Competition

Well, thatās a wrap! Until then, | ![]() |

Reply