- The Vision, Debugged;
- Posts
- Shhh... Can You Hear That? GAMA Can👂
Shhh... Can You Hear That? GAMA Can👂
PLUS: A fill-in-the-blank formula for your dream job transition

Howdy fellas!
It's such a drag... when Spark can't decipher Trouble's mumbled musings. But what if AI could not only understand the context behind every whisper, but reason about it too?
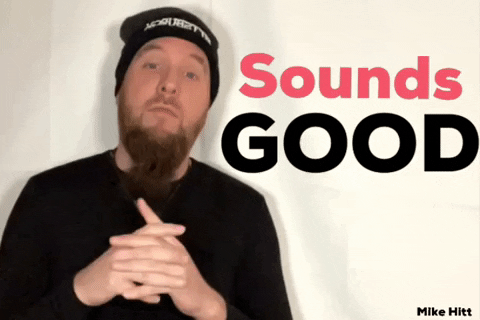
Gif by metahitt on Giphy
Get ready to tune in to a breakthrough that's music to our ears – and brains.
Here’s a sneak peek into this week’s edition 👀
🔉GAMA: The Next Big Leap in Audio-Language AI
🏢 A 2-minute prompt that can help you transition into your dream role
✨3 kickass AI tools that you JUST can’t miss!
Time to jump in!😄
PS: Got thoughts on our content? Share 'em through a quick survey at the end of every edition It helps us see how our product labs, insights & resources are landing, so we can make them even better.

Hot off the Wires 🔥
We're eavesdropping on the smartest minds in research. 🤫 Don't miss out on what they're cooking up! In this section, we dissect some of the juiciest tech research that holds the key to what's next in tech.⚡
Imagine you're editing a home video. You want to automatically add captions describing the various sounds – the roar of laughter, the clinking of glasses, the pitter-patter of rain.
Current technology can handle some of this, but what about the subtler sounds? The sigh of contentment after a delicious meal, the tension in a hushed conversation? These non-verbal cues are crucial for understanding the full picture.
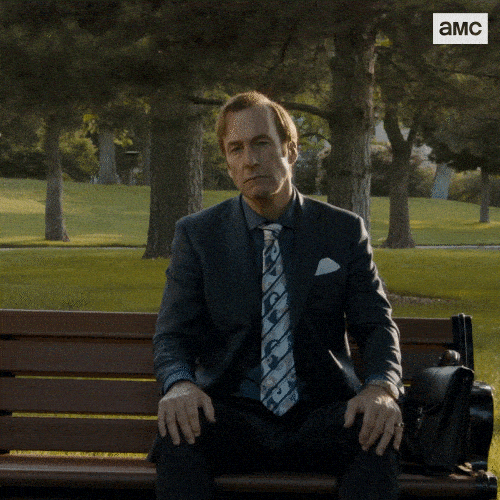
While we've made incredible strides in speech recognition, the realm of using AI to understand non-speech audio remains a frontier ripe for exploration.
Did you know?
The global smart speaker market is expected to reach a whopping $43.3 billion by 2027, and these devices rely heavily on understanding natural language.
Enter GAMA (General-purpose Large Audio Language Model with Advanced Audio Understanding & Complex Reasoning Abilities).
Okay, we get it, the acronym may not be the best one, but this groundbreaking model, developed by researchers from the University of Maryland and Adobe, is pushing the boundaries of audio understanding. GAMA can decipher the nuances of everyday sounds and use that information to reason about the world around it.
Understanding the ‘Jargon’
Before diving into the intricacies of GAMA, let's prep ourselves by unlocking some key concepts:
Complex Audio Reasoning: Unlike simpler tasks like audio description or basic question-answering, complex reasoning involves understanding the entire audio scene, including individual sounds and their context, to draw nuanced conclusions.
Audio Spectrogram Transformer (AST): Audio can be ‘visualized’ when converted into a spectrogram (roughly, think about the waveforms you see on your phone when you record something). AST is a neural network that processes these spectrograms with each layer capturing various sound details. Middle layers focus on basic sounds and textures, while deeper layers delve into intonations and the overall soundscape.
Q-Former: Short for ‘Querying Transformer’, this architecture is borrowed from the vision domain. Think of it as the auto-focus system in a camera - while the entire image is being processed, the Q-Former actually learns a way to “query” or zoom into specific parts of the image & understand details in the scene. Check out this article for an in-depth understanding of Q-Formers. A similar approach can be used to align other multimodal inputs as well.
Contrastive Pretraining: The crux of this method involves a process where the model learns to distinguish between related and unrelated pairs of multimodal data, such as images and text. The model is taught to maximize agreement between representations of similar concepts across the modalities, while distinguishing between the non-related concepts.
Soft Prompting: Think of this as nudging the LLM with a dynamic hint based on the input to ensure better responses
Low Rank Adaptation (LoRA): This approach has been the talk of the town lately - it’s a great way to optimize the training of these massive LLMs by tweaking tiny parts of it by using approximations, instead of the whole thing. Want to understand the nitty-gritty details? Check out this Medium article.
Okay, that might have been a little intense for a pre-requisite, but we’re now reasonably equipped to appreciate the novelties in GAMA.
So, what’s new?
The journey of Large Audio-Language Models (LALMs) has seen significant milestones.

A timeline of large audio models (source: LAML survey paper)
Models like SpeechGPT & AudioPaLM have advanced speech recognition and comprehension. Innovations such as AudioLM & AudioGen have pushed the boundaries of audio generation. MusicLM and MusicGen have revolutionized how we create and interact with music. Models like LTU (Listen, Think, Understand) and SALMONN (Speech Audio Language Music Open Neural Network) have broadened the scope of audio interpretation.
Despite these advances, current LALMs face limitations. Most models use single connection modules between audio encoders and language decoders, hindering comprehensive multimodal connection. Real-world audio, often comprising multiple overlapping acoustic events, poses a significant challenge.
Furthermore, while existing models excel at basic tasks like audio description and event detection (for example, ‘How many doorbells did you hear?’), they struggle with complex reasoning, like inferring activities based on subtle audio context & establishing potential relationships between the entities identified in the audio.
GAMA has been carefully developed to address these shortcomings & take audio-understanding to a whole new level!

Contrasting GAMA with the existing LALMs (source: GAMA paper)
Under the hood…
While GAMA relies on the AST (audio spectrogram transformer) to extract various features from the audio clip, its architecture tries to move away from a single module to feed these audio features into the LLM by intelligently incorporating a multitude of auditory information, obtained using different modules, to capture several acoustic nuances:
Multi-Layer Aggregator: Imagine having access to both a child's simple description of a sound and a sound engineer's technical breakdown. This module examines features from various AST layers to gather a comprehensive picture of the sound.
Audio Q-Former: Inspired by the Q-Former in BLIP-2 for image-text alignment, this component is specifically trained to bridge the gap between audio and language.
Note: Due to the lack of large-scale audio-caption pairs, researchers used the “caption augmentation” method to rewrite captions using different prompts - this helped the Q-Former model learn various distinctive characteristics of the audio concepts corresponding to the acoustic event.
Soft Prompting with Audio Classification: GAMA doesn't just listen blindly. It uses initial audio classifications to guide its deeper analysis. Basically, the result of the AST is run through a classifier to adaptively select appropriate audio event tags. This information is supplied as a “hint” to a prompt template.

Architecture of GAMA (source: GAMA paper)
Before passing audio features to LLM (folks use LLaMA 2 for the implementation), the outputs from each of these modules are passed through respective sets of neural networks, before being concatenated & prepended to the embeddings of the text prompt.
The researchers didn't stop at building a clever architecture - they devised a multi-stage training strategy as well, that greatly enhances GAMA’s capabilities. It is initially fine-tuned on the OpenAQA dataset for audio understanding, as well as some other datasets, to improve its music understanding capabilities. To optimize the training, only the audio encoder & LoRA modules of LLM are trained. Next, it is instruction tuned on the novel CompA-R dataset to endow it with complex reasoning abilities.
What’s the intrigue?
We mentioned that GAMA was instruction-tuned on the CompA-R dataset. But what on earth is this dataset?
CompA-R (Complex Audio Reasoning) is a novel dataset synthesized by these researchers, designed to teach GAMA complex reasoning skills. This involved a multi-stage process, starting with samples from the AudioSet-strong dataset:
Stage 1: The audio & a bunch of details extracted from its corresponding video are used to generate a caption using GPT-4
Stage 2: The captions & actual acoustic event info are passed to GPT-4 to synthesize instruction-response pairs based on few-shot examples. ~25k such pairs are obtained overall
Stage 3: Quality evaluation through expert human verification for a subset of the synthesized data, to form the CompA-R-test set

Staged pipeline to synthesize CompA-R dataset (source: GAMA paper)
The result? GAMA-IT (the instruction-tuned version) outperforms baseline models in tasks like audio classification, dense captioning, and complex audio question answering.
Why does this matter?
Not only did GAMA outperform existing benchmarks, but humans also actually preferred GAMA's responses, finding them more accurate and insightful. You can experience these capabilities for yourself on GAMA’s project page.
GAMA's potential extends far beyond research labs. Imagine a world where:
Accessibility tools that provide rich audio descriptions for visually impaired users.
Security systems can distinguish between a break-in and a cat knocking over a vase, and describe complex audio scenes, like "sounds of glass breaking followed by multiple footsteps.”
Autonomous vehicles can react to emergency sirens even in noisy traffic.
Smart home devices can detect a running faucet or a boiling kettle, saving energy and preventing accidents.

Range of smart home audio devices (source: pandaily.com)
For companies like Adobe, the integration of GAMA could revolutionize audio editing software. From automated sound effect tagging to intelligent audio mixing suggestions based on scene context & enhanced audio-to-text transcription for video editing workflows - the opportunities are endless!
As we continue to push the boundaries of audio-language models, GAMA sets a new standard for what is possible, paving the way for more sophisticated and responsive AI systems. The possibilities are truly exciting!
Key Takeaways
(screenshot this)
Complex reasoning matters: Moving beyond simple classification to nuanced understanding opens up exciting applications
Multi-module Feature Extraction: Utilizing multiple modules to extract comprehensive feature information is a powerful strategy
Data augmentation creativity: When large-scale datasets are unavailable, creative methods like caption augmentation can be used to train models effectively.

10x Your Workflow with AI 📈
Work smarter, not harder! In this section, you’ll find prompt templates 📜 & bleeding-edge AI tools ⚙️ to free up your time.
Fresh Prompt Alert!🚨
Feeling like your current job is just not your jam anymore?
Thinking about making that leap into a shiny new role?
We've got you! 🚀
This week's Fresh Prompt Alert is here to guide you through a smooth career transition. Learn how to leverage your existing skills, build a solid network, and connect with industry pros like a boss. Ready to reinvent yourself and take the plunge? Dive in below and get started!👇
I currently work as a [specify current role] & am considering a career transition into the field of [specify new field or role].
What steps should I take to smoothly transition while leveraging my existing skills and experiences?
I also want to build a strong network in this [specify industry or field]. Could you guide me on where to start, how to approach industry professionals, and maintain these connections for the long term?
3 AI Tools You JUST Can't Miss 🤩
⚙️ ActivePieces - Securely deploy the easiest automation tool for your marketing, sales, operations, HR, finance and IT teams
✈️ Trip Planner AI - Turn your next trip into a hassle-free experience
🎙️ MakePodcast - Effortlessly Craft Professional Podcasts in Minutes Using AI

Spark 'n' Trouble Shenanigans 😜
Sometime in the future? What do you think?

Before GPUs were cool, our brains did all the heavy lifting 😁

Well, that’s a wrap! Until then, | ![]() |

Reply