- The Vision, Debugged;
- Posts
- Can a Robot Really Beat You at Table Tennis? š Find Out Now
Can a Robot Really Beat You at Table Tennis? š Find Out Now
PLUS: Elevate your product planning with this PRD Prompt

Howdy fellas!
This week, our dynamic duo is diving into something electrifying that promises to ignite your curiosity and fuel your next breakthrough.
Stay tuned as we unravel the secrets behind groundbreaking advancements and how they could spark your next big idea!
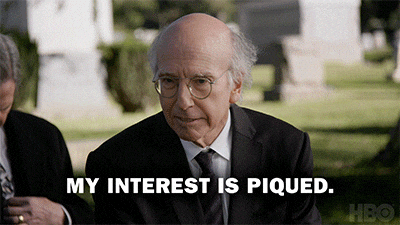
Gif by curbyourenthusiasm on Giphy
Hereās a sneak peek into todayās edition š
Meet the robot that's beating human table tennis players
Struggling with feature requests? This PRD Prompt will save you!
3 awesome AI tools that you just canāt miss!
Time to jump in!š
PS: Got thoughts on our content? Share 'em through a quick survey at the end of every edition It helps us see how our product labs, insights & resources are landing, so we can make them even better.

Hot off the Wires š„
We're eavesdropping on the smartest minds in research. 𤫠Don't miss out on what they're cooking up! In this section, we dissect some of the juiciest tech research that holds the key to what's next in tech.ā”
As the dust settles on the epic 2024 Summer Olympics, you might think the world of sports is taking a breather. Think again! While human athletes recover from their medal-winning feats, a new challenger has stepped up to the table ā quite literally.
Researchers at DeepMind have created a robotic table tennis player that's giving human amateurs a run for their money. Yes, you read that right! A robot thatās achieving human-level competence in playing table tennis, and it might just be the most thrilling development in sports tech yet.

DeepMindās robotic TT player in action (source: project page)
Did you know?
If youāve ever followed RoboCup, you know about the ambitious vision to develop a team of fully autonomous humanoid robot soccer players capable of winning against the human World Cup champions by 2050.
Now, imagine that same level of determination and innovation brought to the world of table tennis!
Forging the Fundamentals
Before we dive into the nitty-gritty, let's break down some key terms:
Reinforcement Learning (RL): is like training a dog: the AI system learns by trying actions, getting rewards or penalties, and figuring out what works best to achieve its goal. It's all about learning through trial and error in a simulated environment.
Imitation Learning (IL): The AI equivalent of "monkey see, monkey do" ā learning to perform a task by mimicking the actions of an expert. Instead of figuring things out from scratch, the AI system follows the expert's examples to quickly learn the right behavior.
Degrees of Freedom (DoF): The number of independent ways a robot can move. More degrees = more flexibility!
Markov Decision Process (MDP): This is a framework used in decision-making where an agent chooses actions to maximize rewards over time, considering both current and future consequences. The most important assumption here is that the AI makes decisions based on the current state, not the entire history.
Policy: In RL, this is the strategy the AI uses to decide what actions to take. It can be implemented in various ways - in deep RL techniques, policies include deep learning models that take as input the various parameters of the environment & output the best possible action for the AI to perform.
Blackbox Gradient Sensing (BGS): It is a method that helps robots learn better ways to interact with humans by testing and tweaking their behavior in simulations. It gradually improves these interactions by combining lessons from both virtual tests and real-life experiences. For more details, refer to this paper.
So, whatās new?
Robotics has come a long wayāfrom Boston Dynamics' robots performing parkour to those that can cook, clean, and even assist in surgeries.
But mastering the lightning-fast, strategy-packed world of table tennis? That's a whole new ball game. Table tennis isn't just a fun pastime ā it's a treasure trove for advancing robotic capabilities. Here's why:
It requires complex motion and split-second hand-eye coordination
Players need high-level strategies that adapt to opponents' strengths and weaknesses
Success depends on both strategic decision-making & physical execution (unlike purely mental games like chess)
Sure, there are "robots" in table tennis today, but most are glorified ball launchers. Some more advanced models can return balls, hit targets, or engage in cooperative rallies. But a full, competitive match against a human? That's been the stuff of science fiction ā until now.
DeepMind's latest creation is the first learned robot agent to achieve amateur human-level performance in competitive table tennis. Here's what makes it special:
A robotic arm with 6 degrees of freedom (3 for translation, 3 for rotation), sporting a 3D-printed paddle
Two cameras to track the ball's position
A unique approach combining the best of reinforcement learning & imitation learning
The ability to adapt on the fly to human opponents' playing styles
Under the hoodā¦
This robotic table tennis player isnāt just a product of raw computational power. Itās the result of an intelligent blend of reinforcement learning (RL) and imitation learning (IL). The researchers alternated between training the robot in a simulated environment (RL) and then deploying it in the real world (IL), where it could play against human opponents without any additional tweakingāa process known as zero-shot deployment.

Overall schematic of the robot during training & deployment (source: paper)
Table tennis gameplay is modeled as a Markov Decision Process (MDP), where each ball hit and returned is treated as an individual episode. The secret sauce to using this model effectively here is a hierarchical control system:
Low Level Controllers (LLCs): Instead of one monolithic controller, 17 specialized LLCs were trained to handle various aspects of the game, like targeting specific return locations or maximizing return velocity. This modular approach prevents catastrophic forgetting (where the robot might forget how to perform a skill after learning a new one) and allows for quick decision-making.
High Level Controller (HLC): The HLC is the mastermind that decides which LLC to activate in response to each incoming ball. Within just 20 milliseconds, it assesses multiple factors to decide on the best LLC to choose for an episode. The HLCās decision-making process is powered by a combination of convolutional neural networks (CNNs) that first decides whether to play a ball using forehand or backhand, along with a custom spin classifier & an algorithm that updates the preference of each LLC by adapting to the opponentās playing style during gameplay.

Intuitive visualization of the hierarchical control system with LLCs & HLC (source: project page)
Training the LLCs was another intricate task in itself:
The researchers first created a custom table tennis simulation environment using the physics engine MuJoCo
They used the Blackbox Gradient Sensing (BGS) algorithm to train generalist policies for forehand & backhand separately
Each of these generalist policies was refined into several specialist LLCs based on advice from table tennis coachesāa perfect example of how human expertise can enhance AI development
If these specialized LLCs performed as expected during evaluation, they were retained, else discarded
But simulation can only go so far. When deployed in the real world, some corrections were needed, particularly for dealing with topspin and underspin. To address this, the researchers added āadaptersā to the LLCs, ensuring the robot could adapt its learned skills to real-world gameplay.
Why does this matter?
When pitted against 29 human table tennis players of varying skill levels, this robot showed astounding results. Although it struggled against advanced players, it managed to crush beginners (100% win rate) and held its own against intermediate players (55% win rate)!
Donāt believe us? Check out the actual match highlightsš
Not only did the robot demonstrate competitive gameplay, but it also made the experience fun and engaging for human players, which is crucial for wider acceptance and use of such technology.
This isn't just about dominating your local table tennis league (although that would be pretty cool š¤©). Spark & Trouble canāt help but think about the implications of this research stretch beyond the TT table.
Adaptive Robotics: Imagine industrial robots that can quickly adjust to new tasks or unexpected situations.
Human-Robot Collaboration: This technology could lead to more intuitive and responsive robotic assistants in healthcare, manufacturing, and even space exploration.
Sports Training: AI-powered training partners could help athletes analyze and improve their techniques in various sports.
Rehabilitation: Adaptive robotic systems could provide personalized physical therapy, adjusting difficulty based on a patient's progress.
Entertainment: Think beyond simple animatronics ā we could see truly interactive robotic characters in theme parks or educational settings.
While we might not see robots competing in the Olympics (yet), this table tennis triumph marks a significant milestone in the quest for human-level robotic capabilities. So, the next time you're looking for a table tennis partner, don't be surprised if you find yourself facing off against a mechanical maestro.
Wish to dive deeper into the technicalities of this innovation? Head over to their research paper to know the specifics.
Key Takeaways
(Screenshot this!)
Hierarchical Learning: Combining high-level strategy with low-level skills allows for complex, adaptive behavior
Sim-to-Real Transfer: Alternating between simulation and real-world deployment can bridge the reality gap in robotic learning
Modular Design: Using specialized controllers for different skills allows for easier updating and expansion of capabilities

In the Spotlightš„
Last week, we had a super interesting chat hosted by Tejj, an award-winning designer & author, on his podcast Dā Industry - where we delved deeper into:
The basics of AI and LLMs š
The AI landscape in India š§ā¬š©
Exciting research and product developments in India š¬
Challenges and opportunities in the Indian AI space āØ
Watch the full episode on YouTube for all the insights š
Andā¦donāt forget to share your thoughts in the comments š

10x Your Workflow with AI š
Work smarter, not harder! In this section, youāll find prompt templates š & bleeding-edge AI tools āļø to free up your time.
Fresh Prompt Alert!šØ
Ever felt like youāre drowning in feature requests and a sea of user needs, wondering how to wrangle it all into a coherent plan? This weekās Fresh Prompt Alert is here to save the day!
It's like a product manager's swiss army knife, but way cooler. Itās your go-to guide for detailing everything from objectives to timelines, making sure youāre set up for success.
Ready to turn your product dreams into reality? Let's roll! š
As the Product Manager for a [tool/product] in the [niche] aimed at [describe your target audience], create a comprehensive Product Requirements Document (PRD).
[Details of the product (if available)]
Generate this document by covering the following areas:
Introduction: Briefly explain the productās vision, objectives, and scope.
Target Audience: Detail the primary users and their needs.
Functional Requirements: List the essential features and functionalities for MVP and future releases.
Technical Specifications: Outline the technical architecture, data models, and third-party integrations required.
UI/UX Requirements: Describe the user interface design, key user flows, and interaction models.
Acceptance Criteria: Define what constitutes a ādoneā feature or functionality.
Metrics and KPIs: Identify key performance indicators to measure success.
Compliance and Security: Explain any legal or security considerations.
Timeline: Estimate the development timeline, including milestones.
3 AI Tools You JUST Can't Miss š¤©
DataLab - Allow AI to write code, run analysis & share data insights
LlamaCoder - Turn your ideas into an app within seconds
GuideJar - Create step-by-step guides & demos effortlessly with AI

Spark 'n' Trouble Shenanigans š
Spark & Trouble are finally able to dance!šŗš
Check this out š

Spark & Trouble dancing (source: created by authors using AI)
And it was possible all using AI - if you too wish to create something similar, just follow these steps:
Head over to https://sketch.metademolab.com/
Upload any sketch that you wish to animate
Set the ājointsā appropriately when prompted to
And voila! Thereās your animated sketch!
You can choose from a range of interesting actions to animate your sketches.
Do check it out & have fun! š
Donāt forget to send us your creations

Well, thatās a wrap! Until then, | ![]() |

Reply