- The Vision, Debugged;
- Posts
- Your AI Needs This! Discover the Power of External Data
Your AI Needs This! Discover the Power of External Data
PLUS: How to Create a Course Students Canāt Stop Talking About?

Howdy fellas!
Our dynamic duo is diving deep into the ocean of external knowledge, pulling out pearls of wisdom that promise to supercharge your models. But bewareāonce you see what theyāve discovered, you wonāt want to go back! š
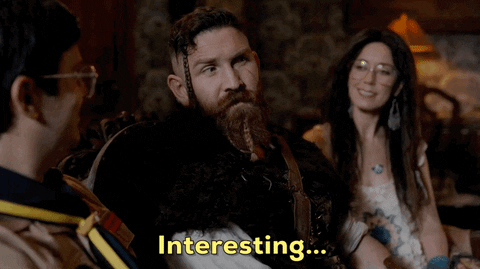
Gif by cbs on Giphy
Hereās a sneak peek into todayās edition š
š¤ Are You Making the Most of External Data in Your AI Applications?
š§āš« Transform Your Course From Dull to Dynamic with This Prompt
š® 5 amazing AI tools that will want you leaving for more
Time to jump in!š
PS: Got thoughts on our content? Share 'em through a quick survey at the end of every edition It helps us see how our product labs, insights & resources are landing, so we can make them even better.

Hot off the Wires š„
We're eavesdropping on the smartest minds in research. 𤫠Don't miss out on what they're cooking up! In this section, we dissect some of the juiciest tech research that holds the key to what's next in tech.ā”
From chatbots that seem to know all the latest tech specs to recommendation engines that understand your preferences better than you do, LLMs are constantly evolving to handle more specific, high-stakes applications. A crucial piece of this puzzle lies in how these models access and use external data, whether to boost accuracy, handle domain-specific queries, or adapt to rapidly changing information.
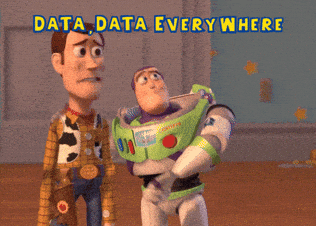
Gif by Giflytics on Giphy
Several of our previous editions have showcased applications of LLMs using various techniques, such as Retrieval-Augmented Generation (RAG), fine-tuning, and dynamic knowledge integration. And weāre sure many of you might be curious to know about:
How can LLMs get even better at using external data?
What exactly are the differences between these techniques?
When to use which technique?
Thatās why when Spark & Trouble came across this recent survey paper from Microsoft Research, they couldnāt resist breaking it down for you!
So, whatās it all about?
External data is crucial for LLMs for several reasons, especially when applied in various fields:
Enhances Domain-Specific Knowledge: External data allows LLMs to access current and specialized information that may not be included in their initial training.
Reduces Hallucinations: By integrating external data, LLMs can provide more reliable and accurate outputs.
Supports Real-World Applications: LLMs need external data to tackle real-world tasks like analyzing financial trends or medical records, helping them make sense of all that complex info.
Improves Interpretability: Using external data helps LLMs explain their answers better, making it easier for users to understand where the information is coming from and trust the modelās responses more.
However, all external data is not the same! Neither are the requirements of different data-augmented LLM applications. The researchers understood this and came up with a clever way to categorize various applications into 4 major buckets (based on the type of query).
These are fairly intuitive & understanding them will enable you to choose the right set of techniques for your use cases.
Forging the fundamentals
Letās get up to speed with some of the terminology that you should be aware of to understand the essence of this research:
Retrieval Augmented Generation (RAG): This is a technique where an AI model retrieves relevant information from a knowledge base and then uses that information to generate more accurate and informative responses, like how a student might look up information in a book to write a better essay.
Fine-Tuning: This is the process of taking a pre-trained AI model and further training it on a specific task or dataset, to make it better at that particular task, like how a professional athlete might fine-tune their skills for a specific sport or competition.
Knowledge Graph (KG): This is like a map of information that connects different ideas, facts, and entities, helping computers understand relationships and context in a more human-like way.
Prompting: This is the art of crafting the right input or question to an AI model, to get it to generate the desired output or response, like how a good question can elicit a thoughtful answer from a human expert.
In-Context Learning (ICL): This is a technique where an AI model learns from the context provided in the prompt, rather than relying solely on its pre-existing knowledge, similar to how a person can learn new things by observing and understanding the context around them.
Under the hoodā¦
Letās now break down each of the 4 categories of queries for data-augmented LLM applications:

Increasing complexity of the 4 levels of queries in data-augmented LLM applications (source: research paper)
Level 1 - Explicit Fact
Level 1 queries are straightforward, fact-based questions like āWhere will the 2024 Summer Olympics be held?ā They donāt require complex reasoningājust the right info from external data.
The challenge for LLMs is making sure they retrieve accurate and up-to-date information quickly. If the data isnāt well-organized or easy to access, the model might stumble.
Solutions? Keep external data well-structured, and regularly updated, and use RAG techniques to ensure your AI always finds the right facts fast! This can include a plethora of modalities (documents, images, videos, web pages, etc.)
Did you know?
There are various ways to optimize RAG applications. One of the most common ways is to perform āchunkingā while ingesting the documents into the database
Level 2 - Implicit Fact
Level 2 queries are like the "brain teasers" for AIāthey go beyond simple questions and need a bit of reasoning and linking facts together. Imagine asking, "Who's the majority party in Canberraās country?" The model first has to know Canberra is in Australia, then find out the leading party.
These questions challenge AI by requiring it to piece together info from different sources, make logical jumps, and avoid misunderstandings.
To tackle this, advanced retrieval techniques like iterative RAG can be used. Here, the model first generates an initial answer based on what it knows. Then, it retrieves additional information to fill in any gaps or correct mistakes in that answer. This process can happen several times, with the model refining its response each time.
Another really cool way to work with such applications is to maintain the external data in a knowledge graph (KG) and use techniques like Graph-RAG to retrieve relevant information optimally from the KG before answering the question.
Since these are multi-step fact-finding type questions, another interesting way to approach such applications is to convert the text query into SQL (hereās a deep dive on several methods to do so) & use that to search over an SQL database of external data.
Level 3 - Interpretable Rationale
Level 3 Queries are the kind of questions where LLMs have to go beyond just giving factsāthey need to explain why something is true. For example, asking āWhy is it important for a drug to follow FDA guidelines?ā means the model has to not only know about the guidelines but also explain their significance.
The challenge here is understanding complex contexts, connecting the dots from multiple sources, and giving a reasoned answer. Moreover, since the context here could be fairly long, optimizing the prompts for LLMs to answer such questions is of great importance.
This is exactly where techniques like prompt tuning & chain of thought (CoT) prompting shine.
Prompt tuning adjusts how prompts are phrased to help LLMs better understand Level 3 queries. By refining instructions and offering clear examples, this method enables models to grasp complex contexts and provide more accurate, rationale-driven answers.
CoT prompting encourages step-by-step reasoning, which is perfect for Level 3 queries that require breaking down complex ideas. This method allows models to reason through each part of a question, leading to more comprehensive and well-explained responses.
Level 4 - Hidden Rationales
Level 4 queries are the trickiest questions, and they need super deeper understanding and reasoning skills to answerāthink of asking, "Why do some medications work better for certain patients?"
To tackle these, LLMs face challenges like making connections from hidden data and needing complex reasoning skills, along with a lack of precise documentation to infer the results correctly.
This is where techniques like in-context learning & fine-tuning come in handy. Offline learning is another interesting paradigm to address level 4 queries. It includes techniques that are used to improve the performance of models by learning from past data without needing real-time input. Here are some key methods:
Guideline Extraction: Learns from past data to create rules, helping the model avoid past mistakes and make better decisions.
Iterative Few-Shot Learning: Starts with a few examples and gradually learns to generate more, similar to improving skills through practice over time.
Error Analysis and Generalization: Focuses on identifying and understanding errors to create general rules, allowing the model to learn from both successes and failures.
Hierarchical Clustering: Groups similar errors to recognize patterns, enabling the model to develop strategies to avoid repeating those mistakes.
Experience Accumulation: Gathers experiences from different tasks to inform future decisions, much like how people learn from their past experiences.
Why does this matter?
Although this may not be a typical ābreakthroughā in AI that Spark & Trouble discussed today, this understanding is essential for advancing the field of natural language processing and improving the utility of LLMs in various domains.

Specific techniques to effectively build LLM systems to address specific levels of queries (source: research paper)
Internalizing this classification of queries & the methods to address them can enable AI enthusiasts like you to quickly & correctly choose the right frameworks to build your own AI applications that rely on external data.
Our resident data enthusiast (aka Trouble) would be curious to learn more about your endeavors in this space to assist you in any way possible.
Write to us about whatās on your mind and how might you use these techniques in your projectsā¦

10x Your Workflow with AI š
Work smarter, not harder! In this section, youāll find prompt templates š & bleeding-edge AI tools āļø to free up your time.
Fresh Prompt Alert!šØ
Ready to share your genius with the world but stuck wondering if anyone will actually stay awake through your course? Been there!
This week's Fresh Prompt Alert is your personal instructional design sidekick! It helps you turn your brain dump into a binge-worthy learning experience. It's like having a veteran teacher whispering the perfect lesson plan in your ear.
Ready to be the instructor students rave about? Take the prompt for a spin š
Act as a seasoned course instructor in the field of [domain], proficient in curating top-notch curriculums that students enjoy.
Design an engaging online course on [topic], with personalized learning experiences.
Customize content, pace, and assessments to optimize student outcomes. Leverage interactive multimedia and real-time feedback for maximum engagement.
Blend self-paced and instructor-led activities to support diverse learning styles.
Need inspiration?
Check out how we obtained a stellar course outline based on an international bestseller:
3 AI Tools You JUST Can't Miss š¤©
š timeOS Magic Notepad: Organizes your meeting notes and action items while you focus on the conversation
š£ļø Aptitude: Let AI conduct and analyze conversations with hundreds of customers
š Langtail: The low-code platform for testing AI apps to catch bugs before users see them
š¼ļø OmniGen: Consistent AI Image Generator Online
ā” Bolt: Prompt, run, edit, and deploy full-stack web apps

Spark 'n' Trouble Shenanigans š
Our dynamic duo is here with a meme that truly captures the sentiments of some users after playing around with OpenAIās much-coveted āking-of-reasoningā o1 model.
(Check out this Reddit thread for more context)
Well, looks like there is still a long way to go for AI models to be hallucination-free!


Well, thatās a wrap! Until then, | ![]() |

Reply