- The Vision, Debugged;
- Posts
- How Chinese Researchers Just Democratized AI Web Agents with WebRL
How Chinese Researchers Just Democratized AI Web Agents with WebRL
PLUS: Your digital twin is just 5 steps away...
Howdy fellas!
Spark and Trouble are diving headfirst into the wild world of online agents, where every click, scroll, and search leads to smarter, more adaptable AI.
Buckle up as they explore how digital minds are evolving to keep up with us all!
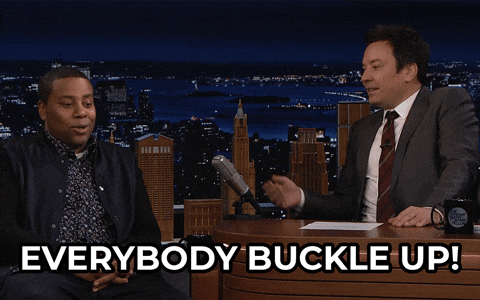
Gif by fallontonight on Giphy
Here’s a sneak peek into today’s edition 👀
💻 Meet WebRL: The self-evolving AI that's making web navigation effortless
📄 Who needs a research assistant when you've got this week's powerful prompt?
🧰 5 super-cool AI tools aimed to save loads of time
🧑🤝🧑 Quick tutorial to help you create your digital twin with AI
Time to jump in!😄
PS: Got thoughts on our content? Share 'em through a quick survey at the end of every edition It helps us see how our product labs, insights & resources are landing, so we can make them even better.

Hot off the Wires 🔥
We're eavesdropping on the smartest minds in research. 🤫 Don't miss out on what they're cooking up! In this section, we dissect some of the juiciest tech research that holds the key to what's next in tech.⚡
Ever found yourself juggling multiple browser tabs, clicking through endless menus, or find the best deal on a flight?
We've all been there - spending precious minutes (or hours!) on repetitive online tasks that feel like they should be simpler. 🤔
Did you know?
Studies show that people spend an average of 24 hours per year just filling out online forms!
What if you had a smart assistant that could navigate websites for you, understanding exactly what you want and getting it done efficiently?
While companies like OpenAI and Anthropic have shown impressive demos of AI web agents using their proprietary models, creating truly capable web agents has remained a challenge, especially for open-source AI models.
But here's the exciting news: researchers from Tsinghua University and Zhipu AI have just introduced "WebRL" - a groundbreaking framework that's revolutionizing how AI agents learn to navigate the web.
What makes this special? It's the first systematic approach that enables open-source language models to become highly capable web agents through self-improving reinforcement learning!
Forging the fundamentals
Before we dive deeper, let's break down some key terms:
Web Agents: Think of these as AI-powered digital assistants that can understand your requests and navigate websites to complete tasks - whether it's booking tickets, comparing prices, or filling out forms. Unlike simple chatbots, they can actually interact with web elements and perform actions across different websites.
Reinforcement Learning (RL): This is how AI learns through trial and error, much like how we learn to ride a bike. The AI gets "rewards" for successful actions and "penalties" for mistakes, gradually improving its performance.
Curriculum Learning: Imagine teaching a child mathematics - you start with basic addition before moving to multiplication and algebra. Similarly, curriculum learning trains AI models by gradually increasing task difficulty.
WebArena: This is like a training gym for web agents - a specialized environment where they can practice various online tasks across different websites (from Reddit to OpenStreetMap) in a controlled setting.
KL Divergence: It is a way to measure how one probability distribution differs from another. Think of it like comparing two maps: KL-Divergence tells you how different the two maps are in terms of the information they provide. Lower values mean they are more similar, while higher values mean they are more different.
So, what’s new?
Traditional approaches to creating web agents have faced several challenges:
They rely heavily on expensive proprietary models like GPT-4
They need extensive human-written prompts and instructions
They often fail at complex multi-step tasks

Example of web navigation tasks performed by LLM-based Web Agents (source: Conversational Web Navigation paper)
WebRL tackles these challenges through a clever self-evolving curriculum approach. Instead of being told exactly what to do, the agent learns from its own experiences, gradually taking on more challenging tasks as it improves.
Under the hood…
The WebRL framework works through several innovative components:
Self-Evolving Curriculum Learning
The core of WebRL’s success is its novel curriculum learning strategy that automatically generates new tasks based on the agent's previous failures.
Here, each phase builds on past challenges, recycling failed tasks as “seed” instructions for new learning scenarios. These tasks are then filtered by a “critic model,” ensuring they are both challenging and achievable, helping agents continually expand their skills.
Outcome-Supervised Reward Model (ORM)
To simplify feedback, ORM gives straightforward, binary responses (“YES” or “NO”) on task success, making it easy for the agent to understand its progress. No more confusion about partial success - the feedback is crystal clear, helping the agent learn faster and more effectively.
Continuous improvement depends significantly on the effectiveness of the ORM. To validate the efficacy of the ORM, researchers evaluated it against other baseline models to observe that ORM surpasses them, with accuracy of 80%!
KL-Divergence Policy Update
Stability during learning is paramount, and the KL-Divergence policy update achieves this by regulating policy changes, reducing erratic shifts that might hinder performance. By balancing exploration with retained knowledge, the agent can consistently improve without forgetting past successes.
Experience Replay Buffer
This component enables agents to revisit and learn from previous experiences, much like a human would reflect on past actions.
Through “Action Confidence Filtering,” the agent also retains only high-confidence actions, minimizing the risk of overfitting to unreliable data.

Overview of the WebRL framework showing its key components (source: WebRL paper)
The results? They're impressive!
When researchers put WebRL to the test, they found that:
1. Open-source models (Llama 3.1 with 8B parameters) trained with WebRL achieved a 42.4% success rate on complex web tasks - significantly higher than previous approaches (~30%)
2. For tasks requiring 10 or more steps, WebRL maintained a >60% success rate, while other methods dropped to around 20%
3. Even more exciting, when scaled to larger models (like Llama 3.1 with 70B parameters), WebRL achieved a state-of-the-art 49.1% success rate
Why does this matter?
This breakthrough has massive implications for both technology and society:
Democratizing AI Capabilities: Until now, effective web agents were the domain of tech giants with proprietary models. WebRL changes this by enabling open-source models to achieve competitive performance, making this technology accessible to smaller companies and developers.
Real-World Applications: Imagine these scenarios:
E-commerce platforms using web agents to automatically track competitor prices and adjust their own
Travel agencies deploying agents to find the best flight and hotel combinations across multiple websites
Healthcare systems using agents to automatically update patient records across different portals
HR departments automating job posting across multiple platforms while maintaining consistency
Accessibility Enhancement: For users with disabilities, web agents could significantly improve internet accessibility by handling complex navigation and form-filling tasks that might otherwise be challenging.
Curious to know more about WebRL?
Check out the full paper for all the nuances.
Try out the code for yourself using this GitHub repository
As this technology continues to evolve, we might soon find ourselves spending less time on repetitive online tasks and more time on what truly matters.
Spark & Trouble are particularly excited about trying out some WebRL-powered shopping assistants during the next holiday season! 🛍️

10x Your Workflow with AI 📈
Work smarter, not harder! In this section, you’ll find prompt templates 📜 & bleeding-edge AI tools ⚙️ to free up your time.
Fresh Prompt Alert!🚨
Ever felt like you're drowning in a sea of research papers, desperately trying to make sense of who said what and when?
This week's Fresh Prompt Alert is your academic life vest. Whether you're tackling a thesis or diving into market research, this prompt transforms the chaotic paper chase into a structured masterpiece.
It's like having a PhD mentor in your pocket! Do try it for yourself 🔽
Act as a graduate student in a [specific field].
You have been tasked with writing a literature review for a research project. Your literature review should provide an overview of the existing research on a [specific topic] and identify gaps or areas where further research is needed.
Your literature review should include at least 10 peer-reviewed sources, published within the last 5 years, and you should critically evaluate and synthesize these sources to build a cohesive argument. Your literature review should be structured in a clear and logical way, with subheadings to help organize your ideas. Additionally, you should provide an explanation of the methodology used to search for and select sources. Finally, your literature review should adhere to the style guidelines set forth by your department or discipline.
5 AI Tools You JUST Can't Miss 🤩
💡 Archie: Go from idea to production-grade software application 10x faster
🪄 FinetuneDB: AI Fine-tuning Platform to Create Custom LLMs
🫰🏼 SnapCode: Transform images into Code with AI
🦁 RenderLion: Transform Links, Words, and Photos into Animations
📈 Truva: Supercharge your sales team with the power of AI

Spark 'n' Trouble Shenanigans 😜
Spark's been pestering Trouble to help her create digital avatars. After Trouble finally caved in (and secretly admitted it was pretty cool), they spent the whole afternoon turning themselves into digital doubles!
So, here's a super-simple guide to creating your own AI twin using HeyGen:
Hit up HeyGen's website & grab those sweet signup credits
Dashboard → Avatar → Photo Avatar → Create (you know the drill!)
Time for your close-up! Upload 10+ clear, front-facing shots (full body pics work best)
Hit "Train model" & let AI do its magic ✨
Sprinkle in details like age & model type
Now for the fun part: Drop your digital twin into any scene you fancy!
Pro tip from Trouble: The more photos you feed it, the more "you" your avatar becomes! Perfect for jazzing up your presentations, tutorial videos, or just freaking out your colleagues!
Here’s a small sample that we could generate…
The way it says the name might be a little embarrassing 🙈, but the quality is fairly decent. We’re certain that the above pro tip could go a long way improving such videos!
We’re excited to see what you create. If you happen to generate some awesome digital twins, then do share them with us!

Well, that’s a wrap! Until then, | ![]() |

Reply